Understanding BGR vs DVE: A Comparative Analysis
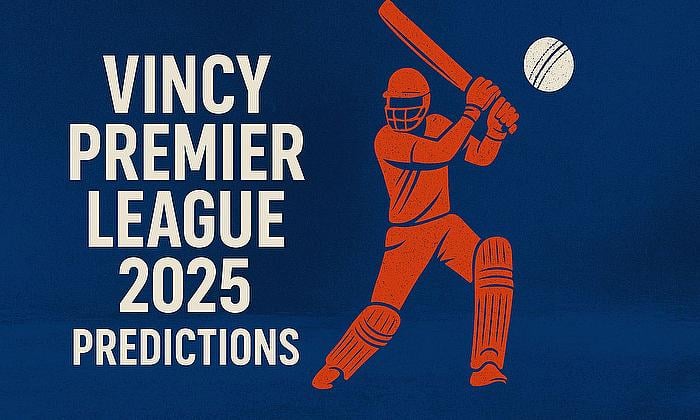
Introduction
The rapid advancement of technology and analytics has led to the emergence of various methodologies for data processing and management. Among these, BGR (Batch Gradient Recovery) and DVE (Dynamic Variable Encoding) are two critical concepts that often surface in discussions of data analysis and machine learning. Understanding the distinction between BGR vs DVE is vital for data scientists and analysts as they seek to optimize performance and achieve accurate results.
What is BGR?
BGR, or Batch Gradient Recovery, is a technique primarily used in the training of machine learning models. This approach focuses on optimizing the learning process by adjusting weights based on the average gradient from a batch of training data, thereby improving efficiency in scenarios with large datasets. BGR is particularly beneficial in cases where the cost of updating the model after every single data point is too high. Recent studies have shown that BGR can lead to faster convergence and improved accuracy under certain conditions.
What is DVE?
Dynamic Variable Encoding, or DVE, represents a more sophisticated approach to handling variable data types efficiently in machine learning models. DVE adjusts encoding strategies dynamically based on the incoming data characteristics, ensuring that the model adapts to changes in data distribution. This technique can significantly enhance model robustness, especially in real-time applications where data characteristics may evolve over time. DVE is also notable for its ability to reduce overfitting, as it encourages more generalized learning patterns.
Comparative Analysis: BGR vs DVE
When comparing BGR and DVE, it’s essential to recognize their core purposes: BGR focuses on improving the efficiency of model training via batch processing, while DVE emphasizes adaptive data representation. In practical applications, BGR might be preferable in scenarios with static datasets, enabling faster training cycles. In contrast, DVE would be advantageous in dynamic environments where data constantly evolves.
Conclusion
As industries increasingly rely on data-driven decisions, understanding the differences between BGR vs DVE is critical for optimizing machine learning workflows. Selecting the right approach can directly impact model performance and the quality of insights derived from data. Moving forward, analysts and data scientists should consider the specific needs of their projects, including data dynamism and resource availability, to leverage the strengths of either BGR or DVE effectively.