SSA vs ASA: A Comprehensive Comparison
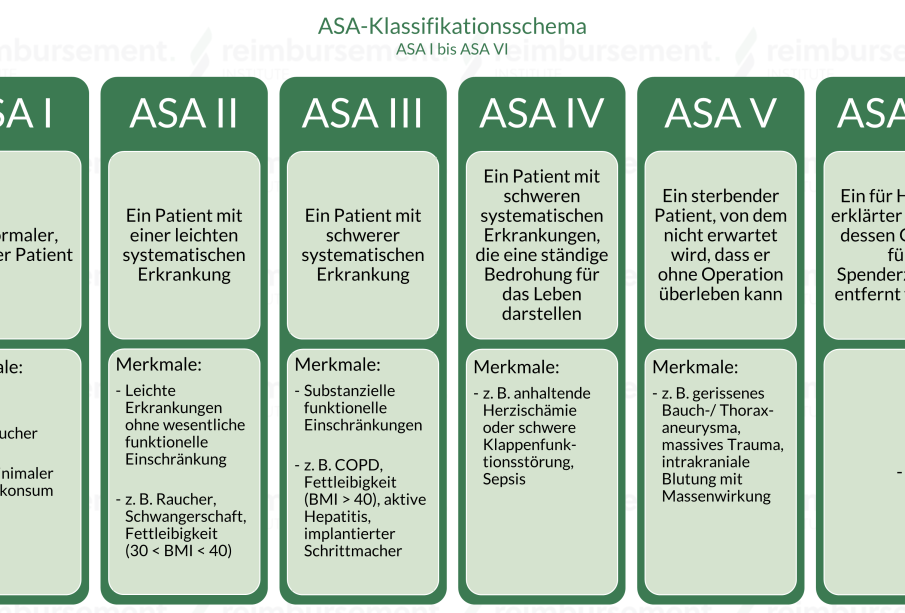
Introduction
The terms SSA (Statistical Sampling Approach) and ASA (Adaptive Sampling Approach) are increasingly prevalent in research methodologies and data analysis. Understanding these concepts is crucial for professionals involved in research, statistics, and data interpretation, as both approaches offer unique benefits and applications. This article delves into the significant differences between SSA and ASA, highlighting their functionalities and relevance in current academic and industrial contexts.
Understanding SSA
Statistical Sampling Approach (SSA) refers to a method where researchers collect data from a subset of a population using random sampling techniques. The primary goal is to infer characteristics about the entire population based on the observations made within the sample. SSA is widely used in surveys, market research, and various fields that require data gathering without exhausting time and resources on studying every single element in a population.
Examining ASA
On the other hand, the Adaptive Sampling Approach (ASA) stands out as a more nuanced sampling technique that allows researchers to adapt their sampling strategy based on preliminary findings. ASA is particularly useful in situations where the data is heterogeneous or the target population is difficult to access. By allocating resources towards areas with higher probabilities of finding relevant information or subjects, ASA increases efficiency in data collection.
Key Differences
One of the most significant distinctions between SSA and ASA lies in their adaptability. SSA is static, engaging a pre-defined method irrespective of outcomes observed during the research process. In contrast, ASA is dynamic, enabling researchers to adjust their approach based on initial observations, ultimately leading to more accurate results and insights.
Applications in Various Fields
Both SSA and ASA have crucial applications in sectors like healthcare, social sciences, and market research. SSA is primarily utilized in settings requiring a representational sample from a finite population, while ASA is applied in complex environments where prior data can inform and enhance sampling outcomes. These methodologies are essential for researchers looking to obtain reliable and relevant information efficiently.
Conclusion
In conclusion, while both SSA and ASA offer robust frameworks for data sampling and analysis, their applicability depends on the research objectives and the nature of the subject matter. As fields evolve and data collection becomes increasingly sophisticated, understanding the nuances between these two approaches will allow professionals to adopt the best methodologies for their specific needs. A judicious choice between SSA and ASA can significantly impact the accuracy and efficiency of research outcomes.