KF vs JF: Techniques That Shape Industries
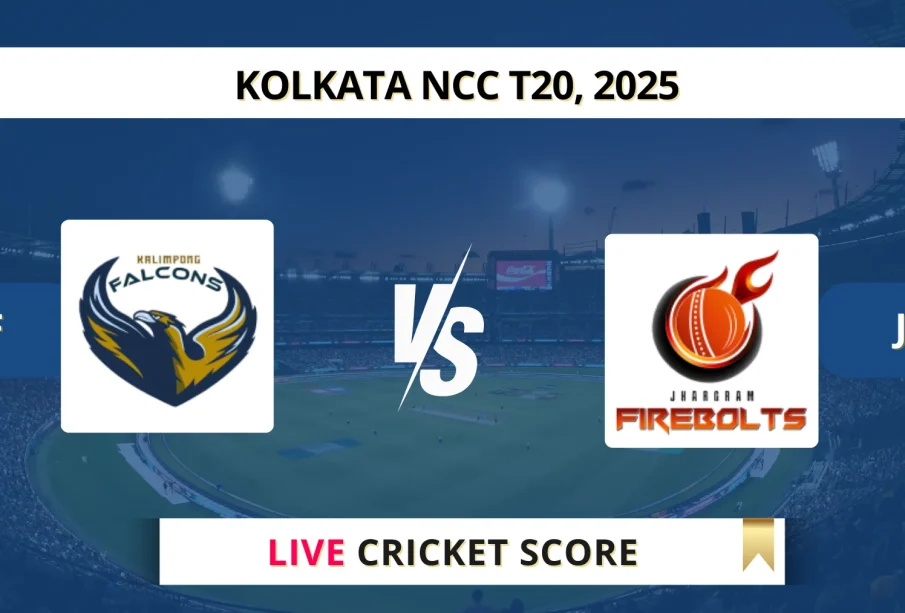
Introduction
In today’s rapidly evolving technological landscape, the debate over KF and JF techniques is gaining traction in various industries, particularly in data processing and manufacturing. Understanding the nuances between these approaches is crucial for professionals aiming to optimize their operations and outcomes.
What is KF?
KF, or Kalman Filter, is a mathematical algorithm that provides estimates of unknown variables. It is particularly useful in predicting the future state of a system based on previous data, applying continual corrections as new data comes in. This technique is widely used in applications such as navigation systems, autonomous vehicles, and financial modeling.
What is JF?
JF, or Jumping Fitness, on the other hand, refers to a form of exercise that incorporates jumping movements, focusing on cardiovascular health and muscle toning. While it might seem unrelated to the KF method, the term JF can also reference specific projection techniques in data analysis that facilitate engagement in physical exercises and health tracking.
Applications and Differences
The primary distinction between KF and JF lies in their application and functionality. KF provides a quantitative method for refining predictions, making it vital in fields that rely heavily on precise measurements and estimations, such as robotics and aeronautics. Conversely, JF’s interactive approach emphasizes user engagement, which can be essential in fitness and health industries.
When comparing the efficiency of these methods, KF is often deemed superior in accuracy but requires more computational resources. JF, meanwhile, can be more straightforward and accessible but may sacrifice some degree of precision in its predictions or insights.
Recent Developments
Recently, the integration of machine learning techniques with KF has emerged as a trend that further enhances its capabilities, allowing for better predictions in more complex environments. Developers are also working on improving user interface designs for JF methods, making it easier for individuals to track their fitness and health metrics through visual data representation.
Conclusion
The comparison between KF and JF illustrates the broader theme of balancing precision and accessibility in technology and fitness practices. As industries continue to develop, professionals must stay informed about which techniques best serve their needs. The ongoing advancements in both fields suggest a future where machine learning and user-centric design will intersect, providing revolutionary benefits in processing data and maintaining health. Consequently, understanding and utilizing both KF and JF will serve as key elements for success in their respective domains.